How to build a data team to uncover retention drivers & fuel growth for your business
Harini Karthik
|
Head of Data
of
Loom
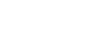
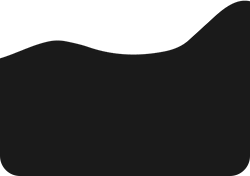
Harini Karthik
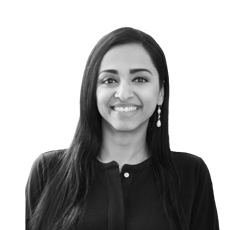
Episode Summary
Today on the show we have Harini Karthik, Head of data at Loom.
In this episode, we talked about the ideal data setup at a company, where to start and how to build a data team that influences strategy and drives growth for the company.
We also discussed why you should prioritize understanding the LTV of your customers as one of your first big data challenges and broke down a step by step guide on how to do it. We dove into how to identify where your users are coming from, the tech stack you’ll need, and lastly how to set up your historical revenue tables for analysis and building out dashboards.
Harini Karthik
Recommends
Customer Churn for MarketersTurning data into insights: A deep dive on attribution and engagementMentioned Resources
Transcription
Andrew Michael: Hey, Harini welcome to the show.
Harini Karthik: [00:01:33] Thank you so much, Andrew,
Andrew Michael: [00:01:35] it's a pleasure for the listeners having he is the head of data at loom leading data science, analytics, and data engineering. Loom allows you to record video messages of your screen cam or both, and it's become a new standard.
And in communications in the workplace prior to loom hernia, as a senior director of data sciences, a chips that was acquired by target VP and head of data at YouCaring, that is acquired good by fund me and the list goes on. So my first question for you hearing [00:02:00] is What has been your process when choosing a new company to work for and how analytical do you get?
Harini Karthik: [00:02:06] Absolutely. I think there are a few key things I always look for. I think one is definitely the product just making sure that it's a product that I really enjoy using. And I made sure that the second thing I would say is just the company and how data-driven the team and the company are, and just the caliber of the talent, because I think, especially with startups in very early stages, Just by the caliber of talent, you can tell whether it's going to be successful.
So I think making sure that I'm always working with people who are really brilliant and that I can learn from that's I would say on the people side, but the being data-driven is key. And then the specifically the role itself I've always looked for a centralized data teams where I've been heading all things, data, and I think that.
It is a very crucial part to me and making sure the data is successful in the organization and that we are using data to help drive growth for the company using data insights or data products. So making [00:03:00] sure that we are a very centralized team, that's serving the needs of the entire company, I think has been very crucial to using data, to drive growth.
Andrew Michael: [00:03:09] Excellent. I'm want to dive into that in a lot more detail in a bit. But it's really interesting what you mentioned as well, like when you choosing company. And the reason I ask is all, because looking at your background, like being at a couple of companies that were acquired, like being at some big brands it's interesting as well.
We recently hosted a rough deli well on the show and he. Also like unbelievable background in terms of the companies have been at like Zendesk, Slack Yammer, like the list was just like, it was extremely long Salesforce, like crazy. How many companies, our kid methodically picked. And the number one thing it came down to is was it a product that he loved?
And did he use it himself? Did he see potential in the product? Interesting. You mentioned as well that the T they like caliber of talent as well, because I think personally as well, that's just the number one critical components as well. How do you assist that? They're going into a company?
Like w what were you looking at? Like, how would, you know if that level of talent is good from the outside?
Harini Karthik: [00:03:59] Yeah, I think [00:04:00] what I look for is really a growth mindset and a collaborative culture. I think that's really the two things I would look for. I'm the growth mindset I'm looking for.
Generally people. Who just loved learning and for them, nothing is too small to do or to learn. They're just always learning and take every moment as a learning opportunity. And I think that growth mindset, I think, is very important that that's something that I value a lot and also very collaborative.
Culture because I personally thrive in harmony and I really think that brings out the creative best in a lot of people. Making sure we're providing a safe environments, I think that collaborative culture, it's something I look for. But beyond that, I think it's, as you're talking to people, especially during interviews, I tried to see, did I actually learn from this person to do, what do I do?
I want to become more like that person. And I think that's the question that I try to always ask myself. It's more me interviewing companies and companies interviewing me, I would say.
Andrew Michael: [00:05:00] [00:05:00] Nice. Yeah, definitely. And I think like you get that sense as well from an interview. Speaking to someone like I, and it goes both ways.
Like when you're interviewing candidates for roles that you have open, or when you're interviewing, you can generally get a sense of the person that you're speaking to. Is this somebody that I feel I could learn from work with and vehicle. Going back into sort of the theme of today, obviously churn fam, we're going to be discussing general attention, but I really wanted to dive into sort of.
For the data science, the data analytics side of things, and how you can set your team up to understand the challenge and the problem. And you mentioned one of the things you look for in a company is typically where data is centralized. And this is one of the areas where you believe like a success happened.
So maybe you can just talk us through the company's thinking about getting data analytics set up at their company. Like what, in your opinion is the ideal setup for data at a company.
Harini Karthik: [00:05:51] Yeah, . I think at a high level of data is a very broad space, but I would say I look at it as three different areas at a high level.
I think one is [00:06:00] more on the analytics side which is helping understand taking a look at the data, analyzing the data, understanding our trends. And then another is data engineering, which is building the data foundation on which analytics can be done, building all the data pipelines. So that data engineering is the foundation on the key and usually a large portion of the data team.
And then and then the third piece is more data science and machine learning. So th these are building data products. And I also call it generally like a data products team, because they're really building more sophisticated models and things that can be integrated into the product itself to drive growth, think recommendation systems and many other things that can happen there.
So I would say these are the three key pillars or domains within data. But. Because we are so highly, cross-functional what I would say the analytics piece actually is very cross-functional because we serve everything. Every team from product to marketing, to sales, [00:07:00] finance, exec, and like support customer service, so many different teams.
And that's where I think we create this little bit more of an embedded model. I would say that where. We are still a centralized team, but we work, we have dedicated resources for each of the other orgs so that they get domain expertise and that they become a thought partner to all our stakeholders.
And that way we can start using data to drive growth rather than being like, Hey, go fetch me this data, or rather than being a report builder, I really see us as more of a. Thought partners. And really, the mission for my team is to influence strategy and drive growth for the company.
So all our projects need to be aligned towards that, but at a high level, that's, those are the three key, I would say data engineering, data, science, analytics, and with an analytics. It's the key to making it work is it creating that partnership and dedicated resourcing for each of the
Andrew Michael: [00:07:52] various organs?
Yeah. It's actually super similar to the way we structured things previously. I was hitting up business solutions at [00:08:00] Hotjar and also a firm believer of having the centralized approach, but distributed at the same time. One of our worries as well was that if you had endless, maybe working out of marketing, and then you had a business intelligence team looking into things, and then you met, perhaps had somebody else.
Embedded in a customer success or so everybody is trying to bring in different analysts is that you never really have that shared expertise. That shared knowledge. You don't have especially when it comes to analytics work, like anybody to bounce ideas off to prove things for you, just like you do code reviews, you do code reviews when it comes to analytics.
So what. What have you found to be the most effective way? Because I think also I think depending on your team size, like at the early stage, perhaps you don't have dedicated resources that you can give to each team. Like, how do you go about starting off in the beginning? Let's like, maybe from the very beginning now you're on 60 70 employees.
Like you maybe might've started a little bit late in terms of data, but you want to get started in your opinion, would it be like the first thing that you [00:09:00] absolutely need to get? And get done from the beginning?
Harini Karthik: [00:09:02] Yep. Absolutely. I think a lot of startups, in fact, when I joined startups, typically it's the team data team is two or three people.
And I helped scale that to 30, 40, and beyond as we grow. But I think that's where it becomes very critical to prioritize and making sure that their stakeholder alignment and what gets prioritized because data teams are in a difficult situation because they're one of the last teams that are formed, but.
The most critical team if you're trying to scale because a lot of decisions need to be made at that point. So I think from that perspective there is that stage where prioritization becomes key. And the way I think about businesses in general, is there acquisition, engagement, and monetization. And I think when we try to look at We just need alignment on where the focus is for the company.
If we're a lot of early startups typically that's on the acquisition side certain companies in more mid stage, maybe shifting focus [00:10:00] to engagement because you want more retention. And then for certain more mature companies, you want to optimize monetization and increased revenue more.
So I think partly depends on the stage of the company, the direction that the CEO and board really wants. One thing is to go, but in a large majority, I think the focus has been very heavy on acquisition. I wouldn't say every company but I would say a pretty good portion because we're trying to grow 10 X, we're trying to go grow big. And I think with that, being a big focus, understanding where are, where customers are coming from and what is which channels are they coming from and which channels are the most driving the highest lifetime value of customers. So I think some of the big prob I think.
One, I would say it is a harder project, but as one of the first projects, I like to prioritize lifetime value of customers. Over time with the ability to slice by which channel they came from, which geography they came from each [00:11:00] product line. So I think that instantly allows you to understand and set, for example, CAC targets for paid marketing.
And it instantly allows us to just understand overall. How we're growing and how healthy our retention is because LTV factors in retention and churn. So I would say it's one of those things where I would definitely say depends on the priorities of the company, but I would definitely say on the acquisition sites, something like, where are users coming from and what's the LTV by.
But the ability to slice by various cuts, I think is very key. On the engagement side of if there are companies focused on that I think it's definitely understanding. What factors drive engagement. And how do you make sure that you can get all users to get to those? So those are some things that come top of
Andrew Michael: [00:11:48] mind.
It's almost identical breakdown in terms of the way we structured things at our charts are very much aligned as well with our company objectives again, sort of thing. We had an acquisition, we [00:12:00] called acquisition retention. Engagement, same thing and monetization. And I think the beautiful thing is like broking the company up into those three pillars.
You can almost every team in the company aligns to those numbers and you can see the inputs that they have that impact the final output. And I think at some point we started getting to like a cascading metrics that trickled down to the organization so that almost every single team in the company could see Whatever it was that they were doing.
How did we impact the metric above them? Whether it was like acquisition, retention or monetization, and then how that sort of winds up the chain. And I think. Getting to that point is a beautiful position because then it's really empowering and teams that can stop thinking about, okay, what are the metrics we need to be moving?
Or like, how do we understand the data and more about, okay, what are the tactics? What are the different ideas that we have that are actually going to move these metrics? I want to dive into the first point though, today. I think it's a perfect topic for the show as well. And I think we can go pretty deep on it as well when it comes to setting up.
Acquisition by LTV and being able to break it down by different segments and being [00:13:00] able to understand where your users are coming from. Because I think ultimately as well being able to acquire the right users and being able to acquire the users with the highest LTV is ultimately going to have a big impact on your attention overall.
Let's break this down for the listeners. Let's take it step by step. You arrive at a new company now there haven't got anything sets up like fresh data stack, maybe two or three people on the team, like you said, and this is the first project you're gonna help the company now get LTV by channel and so forth.
What are the steps? How do you get started?
Harini Karthik: [00:13:32] I think with with LTV I think the first step is to understand make sure that we have two key pieces of data. One is understanding where users are coming from, along with their geo and product line and any other cuts, making sure we have that data available and then making sure that we have revenue data available that can be tied to the user level.
And so I think those are the key ingredients I would say. And the second thing I would say is when it comes to lifetime [00:14:00] value it's a pretty broad and loaded term because there is historical lifetime value and then there's predictive life, lifetime value. I would definitely encourage, let's start with historical wish use what we've already seen.
And then so I think that's that's I would say on LTV and then we want to make sure that we are trying to analyze data at a cohort level, let's take a monthly cohort. So if we want to take Jan 20, 25, 20, 20, and March, 2020 and follow what their, what is their lifetime value month, one month, two month three, and you draw out those curves and then you can start seeing what their LTV looks like.
And then once we have all of these curves at the cohort at the monthly cohort level with the ability to slice. I think a future project can be predictive LTV. We, you have somebody month. One, you're predicting what their one year or two year LTV is. I think this, but I think this is, I think something very important to also make sure [00:15:00] that everybody's aligned on.
What LTV do we also want to use as a break even point? So do we want to break even at a one-year Mark or a six month or one month? And I think that's also like a strategic conversation to also have but I'm happy to dive into more technical details, but yeah. And let me know where you wanna me to dive in.
Andrew Michael: [00:15:19] Let's get technical today then. You started off, first of all, just understanding where the users are coming from. So talk us through this a little bit, like maybe even what tooling you'd want to use to track this. What would you be your ideal tech stack? Perhaps to start and then
Harini Karthik: [00:15:34] yeah, absolutely.
There, so there are a few different ways to approach this. And I, my career I've done it about. Two three different ways. So there's one way of collecting events, data, because a lot of where users are coming from are capture using click events and view events that happen on the front end, which are called client side events.
And those events can be captured in many different ways. One example is So I'm a tool I've [00:16:00] been using for several years now called segment. So segment is a tool that captures a lot of client's side, as well as server side back-end events. And so we use capture all of that within segment.
Another way in the past have done it is Google analytics actually captures all this events data, and they have all their data in the backend which is big query. So you can actually go query the raw data. And so I think that's another way. And then there is also internal tracking.
So segment or not having segment or GA, but you can just have your engineering teams capture all those events, data in house, and then just use that. So I think depending on the, where the company is at, I think people choose different things. And also depending on the business model, because a lot of e-commerce transaction companies, I think Google analytics.
Works perfectly. If you are a subscription model with or like different multi-sided marketplaces. I think like segment or in-house is a better solution. So that's on the tooling side, in terms of [00:17:00] getting the data, I think the neat thing about tools like segment is they're also do all the data integrations for you or a large portion of it, which means that once you capture the events and segment from segment it loads straight away into our data warehouse.
Be it snowflake or Redshift in recent roles I've been using snowflake more, but I think there's Redshift and many others that really can be used in terms of a data warehouse.
Andrew Michael: [00:17:23] Yeah. Yeah. And the beautiful thing as well, I think with segment is that when you capture events through it, it sends it to every downstream destination.
So your CRM in Tacoma will have the same data as a Salesforce, as your data warehouse, everyone's seeing the same numbers, which is beautiful. So I think one of the challenges though When it comes to acquisition in the beginning is understanding, okay, where users are coming from. So you mentioned using the tooling segment itself, but to begin with, you don't know who these users are, like, took us through the process of identifying them.
And then how do you connect the historical history that the user has once they become a user with their past [00:18:00] visits to the site?
Harini Karthik: [00:18:01] Yeah, I think a few different things we do is first off understanding. So within all the segment data that we have, we are able to understand when the user signs up, that's when a user ID is generated.
And, but there's another thing called anonymous ID, which is like a visitor ID which you can trace all their touch points to the very first time they came in. So what that, what we do then is we create a touch table, which is. We create all the different touches that exist for a user until they sign up or convert.
So we're able to then understand what was their first touch, where was their last touch? And we can get into every touch in the middle, but I would highly recommend people start with first touch and last touch. Get that right before diving into other things. So that's, I would say the forest part of understanding where users are coming from.
So you're able to tie the user ID all the way to the channel or the campaign and geo and all the user agent type of things there. And then once you [00:19:00] have the user ID internally being able to map that to activation rates, mapping that to retention rates and then revenue, especially as we're talking about LTV, we want to make sure that we primarily make sure we have.
Revenue at the user ID level over time. So we need to create that sort of a a model there and a table there. And then once you have it, all of the user ID level, you literally join it all. So I know it sounds simple. It generally takes a year for most companies to get this right.
Andrew Michael: [00:19:29] Yeah. So just to recap that as well, like if you're using segment itself as you mentioned that having a concept of anonymous ID.
A visitor visits to a SOC, they get assigned an anonymous ID. The first time they might come back again, like three weeks later. So maybe the first time they came from Facebook, three weeks later, they did a Google search and they came, they visited your site again, that I'm an, a soggy would be fired.
And then the time that they became a customer is when that history would be connected with the anonymous ID, with a user ID. Yeah. Essentially what you said, you have the table then of the different touch [00:20:00] points. And in this case it would just two. So the first and last would be first would be Facebook loss would be Google.
So at that point then now we at least have one part of the equation solve this. We identified users. And now we've connected that black anonymous history with user, you mentioned then being able to have a table then from a historical revenue and what they've spent with you. Talk us through a little bit about.
What stack that you would typically recommend for this? Like how are you pulling this data out? How are you getting a table together?
Harini Karthik: [00:20:28] Yeah. With revenue data. So I think in recent companies we've been using Stripe data for that. With Stripe data, we currently I would say that there, while there are different ways to get it.
So we currently have a couple of tools called stitch data and census that helps pull in some of the Stripe data because we needed at a very granular level. So these are data. These are, again, data integration tools that just help pull the data. Out of the Stripe system. In some cases there are, in some [00:21:00] companies, the the in-house engineering team is able to pull that as well.
So I think there are different comp companies can approach it differently, but the neat thing is there's so much innovation happening in the data integration space that. There are amazing tools out there which have reduced a lot of that effort. So yeah, we that's so we pull that data in and then and then we then stitch all we make sure to have the user ID data attached to the revenue data.
And I think that's that's something that we, that needs to happen. And in some cases that is a challenge. If you are, if. It depends on if you are a very B to C or versus B2B, because with B to C like everybody has an individual subscription. For example, in my past company, I, I was with Shipt every year, every subscription had a user ID tied to it.
But I think with other companies my current company we are, we have individuals, but we also workspaces. It's a very Slack we have workspaces and then we have people within a [00:22:00] workspace as well. And I think th at this point the billing happens at a workspace level, but then revenue happens at an individual level.
So we're trying to see what is the best methodology to allocate a lot of that revenue is I think something that needs to be internal conversations and also, trying to get And puts from the industry, but yeah. So I think that's where getting to that is something that that, that helps eventually get to LTV.
Yeah.
Andrew Michael: [00:22:26] And I think, especially I went in the B2B case when you have a concept of like workspaces and users, like that's when the layer of complexity just gets added onto things. And but I think it can be very interesting as well. I think it differs like wildly, depending on the top of products. I think one thing I've noticed, like when it comes to looking at Different acquisition channels.
If you offer a freemium model, there's not really much difference, but if you have a higher touch sort of sales model where you might have three or four people in a company evaluating your product, they might come over like several weeks from different channels. Then I think things [00:23:00] start to look a little bit different in terms of if you're looking at LTV by channel but.
I think it's, like you said, it could take up to a year to get to the point where you're able to actually understand not only the user level, but also like multi-touch multi-user attribution. And then be able to look into sort of at the account level. So instead of just having user level attribution, you have account level attribution, being able to see those touch points.
So the steps again, we've got now the first anonymous ID is you've identified them accredited a table with the use ideas and the anonymous visitor history. We have a separate table now with the use ideas again, and the historical revenues. How do we tie it all together now? How do we start making use of it?
Harini Karthik: [00:23:41] Yeah, I think now that we have the user ID as the main key that now we can then join it all together. And I'm just for simplicity sake when, and we keep it at the user level here, but yeah, assuming that you have that, then you know that, Hey. Someone coming from this particular campaign and what does their LTV, so we're able to make [00:24:00] that just literally join joined this data set together.
So the end, I think result can be something like a a dashboard that has just. I'm, I'm going to draw it out on the air, but it can have some curves of what does month one through, 30 look like, and by cohort month. So depending on when the user started and making sure that we understand.
What does that LTV that people are coming up with, but in the dashboard, having the ability to select which, which channel you're looking at, which product line, which geo and all of that. And within channel, I think it's important to have first touch and last touch at the very least. So making sure you're able to toggle between, what's initially bringing people to loom versus what's helping convert.
I think those are two. Two big pieces. I think this is, I would say it'd be one, I think eventually where we need to get to with, especially in the B2B space, there's a lot of sales involvement right. In that which I call there's a [00:25:00] sales funnel meets marketing funnel. And I think that's where we need to get to that.
Multitouch of understanding what are those channels, including the sales channel, but the sales channel typically come after or in the middle. We don't want it to get lost. So I would say that's definitely something that we want to focus on as well.
Andrew Michael: [00:25:20] Nice. And yeah, so you mentioned as well then putting together a dashboard, you'll able to see the different cohorts now and breaking them down across different variables.
Like what are some of the interesting things then you've seen teams actually do with these dashboards and how are they used internally? What are some of the most useful cuts you've seen.
Harini Karthik: [00:25:39] Yep. I think one of the biggest use cases for lifetime value is twofold. I would say the first one is It helps us understand how much we can spend to acquire users.
I think that's fundamentally the number one key question. So LTB to CAC ratio, I think becomes important. Because at that point we can [00:26:00] determine, Hey here's the six one-year LTV. And then is that the break even point we want or what break even points should we set and then understanding, Hey, how much should we.
Should we really? What cat should we set? Like how much can we spend to acquire a customer? I would say, and by channel, right? Because what we also see, some channels are actually bringing a higher LTB customers compared to others. Whereas some channels where we're spending a lot, maybe bringing loyalty, big customers.
So there's an opportunity to reallocate spend. So I would say that's where it's everything with Mark paid marketing is a big area that I think. This is critical for and it makes sure it answers a key question of, are we acquiring users profitable profitably? I think that's really the key question we're answering with this LTB.
The I think the other pieces that you also start digging in is. How is LTV changing by different cohorts? Like current, our more recent cohorts have lower LTV, higher [00:27:00] LT. We say, if it has lower, is it because there's more churn or is there an activation problem? I actually feel like LTV can help diagnose issues as well and make sure we're focused on, on, on
Andrew Michael: [00:27:12] some of that.
That's how much an interesting question that I want to ask, because it sounds like as well, choosing a tool like segment. And you mentioned stitch States monuments or the sec, there's definitely a notion of a buy versus bolt. When it comes to your stacks, right? Why would you go ahead then and build something like an LTV dashboard, an LTV model?
Like when tools maybe I think of instruct does give you something out of the box. ChartMogul there's revenue platforms out there that give you sort of these metrics of their what would be the pros and cons of building your own internal model versus using a tool like
Harini Karthik: [00:27:45] yeah, I think using I.
A lot of it depends on the level of sophistication and the business model, right? Because certain business models that have multi-sided marketplaces it, the complexity of the data is very high, where [00:28:00] a lot of these out of the box tools won't be able to easily handle it. Or even if you hack it, it's not gonna last as long.
So I think partly depends on on really the that, and then the other piece of depends on the. Come on the actionability of the data, right? How sophisticated are your questions and how much action are you able to take from those questions and answers? Cause a lot of these out of the box tools may give you high level visibility, but you may, it may not be able to answer the deeper questions and you truly need to solve, helps solve or reduce those problems.
So from that perspective, I would definitely say Building in-house typically is. Has been very important in most roles that I've worked at, including at startups. It's unless you're a very e-commerce transaction focused where you have something like Google analytics that pieces it all together, and you have multi-touch attribution.
I think most, for most business [00:29:00] models that are more complex building it in-house becomes I should pretty critical because you're then able to measure activation you're able to measure churn. And for example in, in my current company at loom activation happens when a different user watches your video, so when you have multiple users that are impacting each other's activation, that's also, an example of why. Out of the box tools break down there
Andrew Michael: [00:29:25] right down. Absolutely. Okay. And then obviously I'm not. And so the question was because I understood the answer, but it's always interesting because of looking at your stack, you've chosen to buy a certain services of building others.
But definitely a, like a C. The point that you mentioned or specifically is that, are you able to actually action on this information and how sophisticated do the questions that are coming through to Jared will give you a quick answer. Is it for you or not? And also the way things going, but definitely a hundred percent agree with you.
Like these out of the box solutions, depending on how complex your product. Like you can only hack them so much until they break. So then. Last [00:30:00] thing I think on this topic just to cover then would be you talked about like historical revenues, historical LTV, you touched on predicting LTV going forward.
Talk us through what that would look like. So you spent the good part of six months, maybe even a year, depending on how sophisticated your team was to get to this point now, and now you to actually start predicting your LTV. So you can actually understand the future revenues and what you can actually start spending from now.
So how do you. Get started you have this model from yeah. Where do you go?
Harini Karthik: [00:30:29] Yeah, I think making sure that we have the historical LTV is I would say the foundation behind getting to a predictive LTV. I think a predictive LTV helps understand, Hey, we're acquiring, we just acquired the user.
What do we think they're worth? And maybe we can predict five years out. And I think that that's something that makes sure that, We understand the value of users and we're shifting focus more real time. And with predicting it's One, as I mentioned, the key [00:31:00] is having that historical data, but we need, I would say a larger historical data or long enough history because a lot of the predictive models need need enough history data.
Yeah, all of the data to be able to predict, because if just to, to simplify it if we look at the different curves and you could actually do this in, in, in Excel as well. If you have a, if you have curves you can I think it will display the equation of the chart of the curve.
So what that does is once you have the equation of the curve then D you can put in whatever value of which month and it'll predict, okay, what is the Y when you have the X, and things like that. So you can pretty easily get to what the predictive value of LTV will be. So I think this is a, I'm just trying to simplify, because what I'm trying to get to is.
You can do very simple manual things in Excel. I think once you want it all an automated and actionable, I think you want to build it in your data warehouse. Make sure you have it in a good dashboarding tool, like Tableau or many others out there. [00:32:00] But yeah, I would say the key is you're trying to find is there any equation.
To my curve or to the historical data. And if there is, we can easily apply that. If there isn't a good one, I think that's where we need to dive deeper into why there isn't a pattern with the data. And that could be because of very little data or it could be because there were a lot of changes made with pricing and packaging.
There could be many other reasons, but I think those are, that's just a high level.
Andrew Michael: [00:32:30] And becomes difficult to predict. Yeah. So that question on this end as well, like in the sense, so you have your curves and you mentioned sort of one way of looking at it just like months that they signed up. Yeah.
And then on previously as well, you talked about like different data points. So like the channel that they came through, like the geo and things like this, when it comes to predictive models and looking at the future looking at LTV would you be segmenting then by these channels again and seeing, okay, what is the future LTV look like for this [00:33:00] cohort?
So if they sign up now it's month, zero for them. You're looking at the attributes then. So you're looking at our kid that came from Facebook that came from this country, and then you're looking at the past performance of that country and that geo and then you're able to predict going forward.
Is that like the simplest explanation?
Harini Karthik: [00:33:16] Yeah, exactly. So a lot, depends on the granularity that you're trying, you have the, your data and then you try to. Piece them together to
Andrew Michael: [00:33:23] try to, and then obviously the more granular you go, the less likely you are to have the data that you need.
Harini Karthik: [00:33:30] I suggest starting with the monthly is because you can go to the daily level.
It's just I think we want to make sure we get the. Bigger picture right before we dive into the details. And with details, I think once you have LTV, you could have things like, Hey, these, this user has high LTV. Maybe we should put the sales team in front of them, or they, or maybe there's a high chance that this will convert better. So I think you can create personalized experiences within the product based on which channel they're coming from [00:34:00] and what their intent maybe.
Andrew Michael: [00:34:02] Maybe and the next question, I think on top of this, just falling into the lands of LTV.
So I think when it comes to acquisition most businesses, like they will see some level of seasonality when it comes to acquiring new customers. So typically like over the December holiday period, you'd see a dip in acquisition and maybe sometime as well, like March, April holidays you see another small dip or summer holidays which is like August I think in Northern hemisphere or not, I think it is.
And I'm interested those all that's obviously one thing acquisition, but when it comes to LTV, do you see similar trends of like months where users are being acquired? Have you seen this in the past or is LTV not really affected by seasonality because it's blended in over time?
Harini Karthik: [00:34:43] I think typically there has been a little less seasonality involvement, just because you're looking at how users or attention over time and while the absolute number of signups may be lower in December and may while while some of the engagement may be lower.
I think if you are, for example, a [00:35:00] subscription-based business the subscription keeps renewing so, or it's so I think that's where it depends on the type of business. I think e-commerce gets hit harder than subscription businesses.
Andrew Michael: [00:35:10] Absolutely. Cool. Jesus, here we are running up on time already.
So I am going to save it for two last questions for today. One question I ask every guest. To the joints to show a hypothetical scenario. Now let's imagine you're joining your company and you're at this company general, attention's not doing good at all. And the CEO comes to you and says, Harini we really need to turn things around.
We have 90 days. To decrease churn and increase retention. It's up to you to solve this. Like you have to save the company. You're not going to do the typical things of going and speaking to customers and understanding the problems and looking at the data. Like you just need to pick one thing from your past that you've seen, that's worked effectively and run with it.
Like what would be that actionable item you'd want to implement at the company?
Harini Karthik: [00:35:57] Yeah, I think I'm churn is definitely [00:36:00] one of the hardest problems to solve because you're trying to change people's mind or behavior. And I think typically it's a very lagging indicator, right? It's not a leading one.
I think with churn and retention, I think one of the. Things that has truly helped. In the past has been understanding the factors for churn and this has been like a churn. Like my team has built churn prediction models in the past which will predict whether user will churn or not. And we use machine learning models to build some of that, but.
I think the key behind it though, is what are the key factors for churn? Because if you understand what are those key. Indications and clues that we see earlier on in their journey that get them there. I think you can try to mitigate that earlier on. So I would say there have been few different things based on some of those churn factors.
Some have been where we have fed in [00:37:00] a lot of this RFM type of segmentation, which is recency frequency and monetary type of segmentation and feed that into your email tools and other tools that can then have personalized messages based on where you are in your life cycle. If you are a dormant, VIP, you may get a different set of communication or emails to try to.
Get you more engaged again. So I would say there was a little bit of that creating that segmentation and understanding who are the people who are going to churn and making sure that you have all the systems in place to make sure you're reaching out to them. I would say that's one. And then again, the other piece would be from the churn factors, making sure that we're addressing it with the product team to make sure that we get ahead of some of this.
But the easier one I would say is probably getting our emails set up.
Andrew Michael: [00:37:47] Absolutely. Yeah. Product changes take time, but like emails or something that you can, if you have the tech stack, can Jeff Good data? I think it's quite an easy one to get out and get up and running. Yeah. Great. So last [00:38:00] question then what's one thing that you know today about chin and retention that you wish you knew you got when you got started with your career.
Harini Karthik: [00:38:06] That it's very hard to move that the reality is I think it, it it took me a few years of experience and some, working with a lot of different teams too, I would say it's definitely a little bit more of that 80 20, where you need to put 80% of effort to get 20% of results. It's, the flipped 80 20 to an extent.
So I think it's definitely, it takes. Oh village to move this mountain. So I think it's a very cross-functional effort between product marketing sales and all the teams to make sure that we are moving this. So it's not a quick experiment and we were, we solved it. So I would say that's the thing is I think it's definitely shows the health of the business and it just takes a while to get there is it's been my learning.
But it's the most important thing to focus on.
Andrew Michael: [00:38:50] I love hearing that as well, especially coming from head of data at a company like loom. And one of the main reasons why I think is because in the beginning, starting out this podcast, like one of the things that frustrated me [00:39:00] was like how we discovered this magical number, the silver bullet, and we all focused on that and we reduce churn and we crushed on numbers and I think.
Whilst you might have a North star metric that is really helpful to get the team to learn around. It's as you mentioned, there's just so many different inputs. It's a lagging metric that's influenced by decisions. Maybe you made months earlier. And really having that, understanding that it's not just like one central thing, that's going to make the difference, but really a considered effort.
And as you mentioned, like it's going to take a village to move the mountain that really takes the whole team to get things moving. So I love that. How do you mean is there any final thoughts you want to leave the listeners with anything you'd like to share today? Like how can they keep up with your work?
Harini Karthik: [00:39:37] Yeah. I think my final thoughts in general is with data keep it simple and treat your data team like your thought partners. And you'd be surprised by how much they're able to help you. That would be my, my key is always keep things simple with data. And yeah, for anyone who wants to reach me, I am on LinkedIn.
My name is. Harini Karthik and yeah, I'm please feel free to [00:40:00] reach out and I'm happy to answer any more questions.
Andrew Michael: [00:40:03] Awesome. Thank you so much for joining. I really appreciate your time today and wish you best of luck now. Going forward.
Harini Karthik: [00:40:08] Thank you so much. It's been my pleasure. Thanks again.
Andrew Michael: [00:40:11] Cheers.
And that's a wrap for the show today with me, Andrew, Michael, I really hope you enjoyed it. And you're able to pull out something valuable for your business to keep up to date with churn.fm and be notified about new episodes. Blog posts and more subscribe to our mailing list by visiting churn.fm. Also, don't forget to subscribe to our show on iTunes, Google play, or wherever you listen to your podcasts.
If you have any feedback, good or bad, I would love to hear from you and you can provide your blend direct feedback by sending it to andrew@churn.fm, lastly, but most importantly, if you enjoyed this episode, please [00:41:00] share it and leave a review. As it really helps get the word out and grow the community.
Thanks again for listening. See you again next week.
Comments
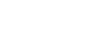
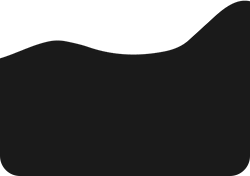
Harini Karthik
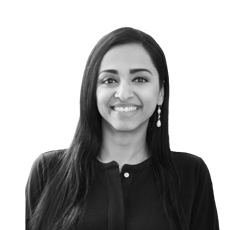
A new episode every week
We’ll send you one episode every Wednesday from a subscription economy pro with insights to help you grow.
About
The show
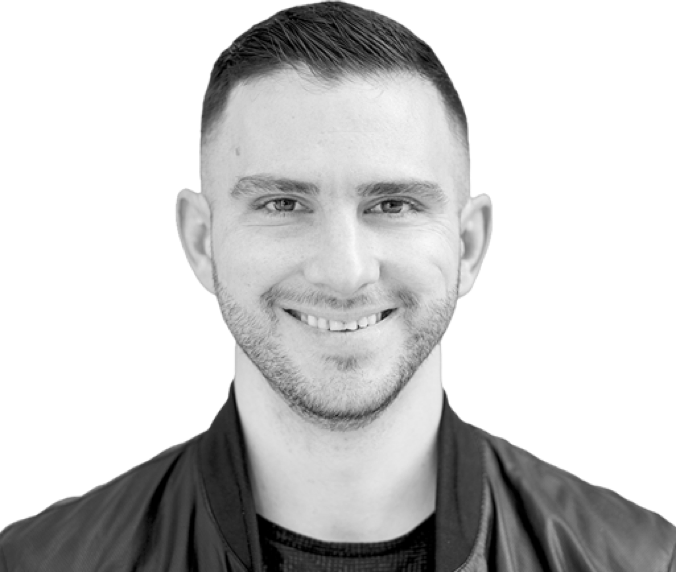
My name is Andrew Michael and I started CHURN.FM, as I was tired of hearing stories about some magical silver bullet that solved churn for company X.
In this podcast, you will hear from founders and subscription economy pros working in product, marketing, customer success, support, and operations roles across different stages of company growth, who are taking a systematic approach to increase retention and engagement within their organizations.