How investment firms use customer behavior predictions to set valuations on the companies they acquire
Daniel McCarthy
|
Director & Co-founder
of
Theta

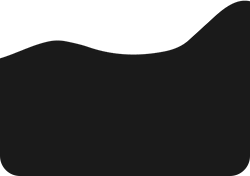
Daniel McCarthy
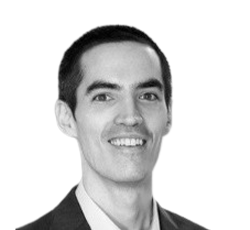
Episode Summary
Today on the show we have Daniel McCarthy, Director & Co-founder of Theta.
In this episode, Daniel shares how he founded two companies all while doing his master’s degree and teaching at a university.
We then discussed the key metrics companies should start tracking to build their evaluation scorecard
And how investors use it to set a company’s valuation and we wrapped up by discussing the relationship between customer acquisition costs and organic acquisition and it’s impact on valuation.
Mentioned Resources
Transcription
[00:01:23] Andrew Michael: Hey, Daniel. Welcome to the show.
[00:01:25] Daniel: Oh, thanks for having me, Andrew.
[00:01:26] Andrew Michael: It's great to have you for the listeners. Daniel is the assistant professor of marketing at Emory university and the direction co-founder of theater, a custom based corporate valuation platform.
Prior to theater, Daniel co-founder Zodiac, a predictive customer analytics platform that was later acquired by Nike and also served as a resident data scientist at Waterton. So my first question for you, Daniel, is it appears from LinkedIn. Both Zodiac and theater. You've also held roles at Emery and Watson university at the same times.
How did you manage that first of all? And do you find that maybe had some sort of strategic advantage while [00:02:00] building those companies with those university placements? Yeah.
[00:02:03] Daniel: It's a really good question. It's a lot of work to both be a PhD student and now a professor while also.
Co-founding a business. I think the main things that have made both of those things work is that for one, the companies are doing the same sort of stuff that I focus on for my academic research. So it's just an area that I spend a lot of time with and really the synergies work in both directions, you're doing all this great academic work in a couple years.
And someone comes to you with a problem about, how to run the numbers on a particular business. And you can draw on that experience that you've had from the models that you built. It can also work in the other direction in the sense that the companies that you work with it really helps understand, like what are the problems that are on the mind of the companies that you're working.
And data sets become not an issue, that you have more than enough data sets that you could ever deal with. And yeah, the question becomes, what is it that you should choose to focus on? I'd say the other thing that's been [00:03:00] extremely important is just the power of knowing what you're good at and what you're not and delegating when when it falls outside of the strike zone.
So at Zodiac, I was the chief statistician I was co-founder and chief statistician. But you, that was kinda all I did was primarily when we had a new company that came to us and, we had to build a model for how customers are going to make purchases in the future. It wasn't captured well by the existing portfolio of models that we had.
It was my job to go in there and specify that new model. And so mobile gaming was one example of that. Just different dynamics than you would see at say an eCommerce retailer. Part of pharmaceutical businesses was another. Telecommunications, we had a large telecom compliant. And so for them, we needed to specify a different model, but for most of the other aspects of the business, I just tried to stay out of the way and for one, I think it's a good thing because it's just not what I'm good at.
And then for two, It allows me to retain that time to be able to [00:04:00] successfully, complete the PhD process and, go and become an academic. Yeah, so it's really been, it's been nice in that way. It's an amazing validation to be able to write these papers for a primarily academic audience and then bring it to life with 200 companies.
But but yeah it's been a lot of.
[00:04:15] Andrew Michael: Yeah. Sounds like an amazing like application as well of the work. And then it's like proving the theory in real life at the same time at the end of it as well, which is great.
[00:04:26] Daniel: We're kinda in the midst of this, they call it the reproducibility crisis. I don't know if you're even following all that, but it's the.
A lot of results within academic literature, don't tend to seem to play out in the real world. And other academics will try and replicate the results of these papers. And they'll say we did it again and we didn't get that . Yeah. And so there's just been this identity crisis that that the whole academic field's been having to some degree.
And this is like the opposite, here, we're taking these methods and we're showing, yeah. You could do it on this business that we [00:05:00] did in our paper, or you could do it on any of the 250 other businesses that we've used it on and it still holds up really quite well. To no, no concerns about generalizability here.
[00:05:10] Andrew Michael: That's great. Yeah, I think cuz that's also like one of the shames I think sometimes is that you hear some great work being done in universities, but then hardly any of it ever gets product ties and actually makes it out past the paper that gets written for it. One like that, it gets out and then two that it's actually working and it's serving great purpose and you validating that over and over is amazing.
I'm interested. You said like you get to do work in a field in a space that you're interested What interests you so much about the space that you're currently in, like custom analytics platform and predictive analytics specifically?
[00:05:41] Daniel: Yeah, I'd say that it's the fact that sits at the intersection of three areas that I just really love statistics, marketing and finance.
I had started on the buy side at a hedge funds. I worked for about six years after I finished undergrad also at warden and And then after that came back for [00:06:00] the PhD, my PhD was formally in statistics, not in marketing, but it was in the second year of the PhD program that someone had connected me with this marketing professor, Peter Fedder.
And and the great thing about this kind of whole area of predicting what customers will do, and then using that to generate insights about how companies as a whole are doing is again, you've got the predictive models, that's all, that's statistics, you're predicting what customers will do, which is fundamentally a marketing problem.
But then you're using that to come to assessments about the overall health valuation of companies, which is a finance problem. And it's very rare to, to find a topic. Hits on all three of those different areas at once. But this is one of the few that does.
[00:06:43] Andrew Michael: Yeah, I definitely see that.
So I'm interested today to dive into a little bit about the new company and theater and what you're doing there. From a very quick skim and understanding as well. It caught my attention, a customer based corporate valuation platform. So if [00:07:00] I'm understanding this correctly, you go in and you help evaluate and set valuations for specific companies.
And you're taking a look at the customers and retention and things like this is this how you go about evaluating C.
[00:07:10] Daniel: Yeah. I'd say that it's actually related to what I had done at Zodiac. So it Zodiac. Yeah, we yeah. So at Zodiac, what we had done was we would take in data from companies themselves, all the transactional and CRM data.
And then we would train a series of predictive models for what each and every individual customer will do in the future. Yeah. How many purchases they're gonna make and how much they're gonna spend when they make the purchases. And then use that to help the marketers make tactical, customer acquisition and retention decisions at data, in some sense, we're using the same models.
But instead of helping the marketers, we're still actually doing that now to to, to a fairly reasonable degree. But the other core constituency has been investors. And So the canonical kind of stereotypical engagement would be we're working with a PE [00:08:00] firm. The PE firm made it past the first round of diligence.
Now there's just a handful of firms. They're looking at this company very seriously. Typically at that stage at the later stage of the diligence process, that company will put more data into the data room and we'll basically train the same models that we did at Zodiac. But now we're using it to understand how good the customer economics are.
How good is customer lifetime value? How strong is the retention? What is the implied marketing return on investment? Those sorts of things and how's that been vary? By acquisition cohort and across different customer segments, you acquisition channel and whatnot.
And then use that to help the PE firm decide. Is this something that we really wanna move forward with or do we see signs that there is some degradation in the most recent say acquisition cohorts that may make us a little bit more hesitant to, to move forward with the deal? Yes, it's really been cool again, very synergistic.
And that's also why we're doing it on the corporate side, but the use cases are just not quite the same.
[00:08:58] Andrew Michael: Yeah, that's interesting. Cause I think if [00:09:00] you torture the data enough, like it confesses to anything in most cases. And I think specifically like in these sorts of larger deals, it is easier to manipulate certain numbers as well, to look better than they actually are at the surface level.
But when you dive a little bit deeper, you start to understand like maybe businesses aren't as healthy as they look. And actually one of the interesting ones, like we talk about a little bit on the show sometimes is very few companies know about what the churn ceiling is. And like when you're gonna hit this growth ceiling due to your churn rates and your growth rates in early stage, like churn is really masked because you have hyper growth and you're growing a lot faster than your churning of customers, but at some points, like it comes to this moment in company's life cycle and they realize, okay, Our growth is gonna stall in like the next 12 or 24 or 36 months, whatever it is.
And then everybody switches on the alarm bells. We need to start focusing on this now because I think having a deep understanding of how the data works and the underlying metrics that actually move the final output, which is churn is really important. Let's dive into this a little bit deep, cuz I think it's interesting for the audience as well to [00:10:00] understand.
And I think from both aspects, one is like, how do you go about understanding this for your business and setting like benchmarks and getting yourself to a point where you have got a good understanding of of how data and your business operating. And then second. How that's gonna influence the overall valuation that you're gonna get into the day and how you calculating that.
I'd be interested dive in that as well. So first of all is as a customer coming to I wanna understand my business better. Like where do you typically get started with these companies and how do you get them set up?
[00:10:30] Daniel: Yeah. Typically, I'll just think of it as like a, it's like a report card. But instead of English and math, it's about customer economics and we'll typically break it down to a series of measures customer lifetime value customer acquisition cost at different retention measures, the expected lifetime of customers.
Yeah. Especially for subscription firms. What it all means for implied marketing return on investment, which is basically, the net present value of the customer divided by, how much had been spent to acquire customers. And yeah, I [00:11:00] think what it can do, if you get those figures, how they've been evolving across acquisition cohorts and for the different segments of your business.
Which you can cut by product of first purchase in store versus online acquisition channel or some other feature. It can really help you understand, like where is the value coming from in your business and which segments of your business are healthy, which are not. And we really recommend starting there and I think what it can do is it can help you get a sense for just how healthy you are.
And And then obviously the next question is what do you do about it? And yeah, I think that's where you can start moving from, purely predicting to being a bit more normative and saying, this segment doesn't seem good, and maybe we can help understand why. And maybe if there's nothing that we can do.
Then we should reallocate budget away from that segment of the business, towards the other segments of the business that seem to be behaving in a better way. So usually starting with that report card and then, seeing what [00:12:00] it implies to decide what the best next step would be.
That makes a lot of
[00:12:03] Andrew Michael: sense. Good place. Yeah. It's actually funny something that I've done very recently. Like last week was busy looking at for my startup as well. Just trying to understand, like now that we've started charging customers and breaking things down into codes, trying to understand how each code is performing.
And I started very similar to what you mentioned as well. I just basically took at like total signups and. By cohort per week, total spend, if anything, in some weeks, a number of customers acquired from their got conversion rates started then looking as well trying to understand what the payback period would be for those customers and see how the cohorts are changing over time.
Unfortunately, we don't have the luxury of server, so many customers where we can go deep into segments yet. , but already starting to see some good signals and good ideas. Like we can make improvements, but. Maybe if we wanna go a little bit technical, like with companies now getting set up and these things together.
So first stage is just getting the report card. As you mentioned, you have your key metrics that you wanna be tracking. You mentioned then [00:13:00] like getting a bit of predictive analytics inside there. What would you typically be looking at then? And how would you be using the inputs that you've gathered from the report card to predict future.
[00:13:10] Daniel: Yeah. So those are part of the report card would be predictive measures. So for things like customer lifetime value, you're gonna have to make a prediction and and predicting is hard. So yeah, I'd say that's where, we fall back on the experience that we've had with the, we probably run the numbers now on 300, 350 distinct customers between the two, businesses that I've started.
And I'd say the easier customers to value are the ones that you acquired a long time ago, because for them you've observed more of their lifetime already. The really hard ones are those young customers that you're acquiring over the past six months. And the tricky part is that those are the customers that are in some sense, most relevant to you, cuz for one, because they're earlier on in the relationship.
They're probably more malleable. If you've been with a firm for five years, 10 years you're probably pretty set in your ways. so not a whole lot that you can change. [00:14:00] And because, if you acquired a customer only, three, three to six months ago, it also means you don't have a whole lot of transactional behavior to make that prediction with.
And so you just have less data, you have more uncertainty in what the future's gonna. And that's really where you want to lean on very good models, that allow you to be able to make those predictions. And so we really pride ourselves in being able to predict very accurately. But we're the first to say that a lot of the value is coming from our ability to connect the dots across the different acquisition cohorts so that we can be able to take those young cohorts and still be able to predict for them reasonably accurate.
[00:14:37] Andrew Michael: Yeah, I think that's one of the challenges as well, like you say, is that LTV itself is the more data you have to predict it the better, but unfor the newer customers are the ones that are coming to the product for, as it is today. And the experience it is today and newer customers in an older company will typically be a little bit more fickle than all the customers, because you tend to get like the crossing of the chasm where you've gone.
[00:15:00] Early adopters who were happy just to get better things. And then later stage, it's funny because like I now just started setting up analytics for ourselves, for revenue and stuff. And I like, I'm not gonna mention any names, but I added two or three different revenue reporting services. And each one of them has reported LTV differently.
There isn't one, that's giving me the same number and they're very, quite a bit like there's a variance of like 10 to 15% between each of them. And they're obviously all using their. Models to calculate because it's the same data that's being fed into each one of them. So interesting. There's so from your side of your perspective, like when looking at LTV, you tend to wait all the customers more in predicting customer lifetime value.
[00:15:40] Daniel: We predicted the individual level and so each customer in that sense gets their own weight. But we wanna learn from all the customers and, obviously the. The older customers can really help us understand what the young customers will do. You it's really it's an important data point.
So in that sense older customers will get, meaningful weight [00:16:00] when we're making the predictions for very young customers, but maybe not so much the other way around.
[00:16:05] Andrew Michael: Yeah. So I wanna try something interesting, if I was an investor, I came to you and said, Hey, Daniel we're looking to invest in this company.
We'd like you to come and let us know is this a good investment that we should be making? What metrics would I be asking you for to see, first of all, and then what would be like really positive indicators and indicators that would get them to question their investment, that you would start to see
[00:16:28] Daniel: Yeah I'm a big return on investment person.
If you're evaluating a capital project ultimately what you care about is, what is its net present value and what sort of return am I gonna get on the invested capital that I put into it? And yeah, I think of the customer in the same way. It's just that now the project is the customer.
And so yeah, in that sense, a lot of our sort of measures would revolve around return on investment. And I'd say the second broad category is about quantity. Having a high lifetime value, having a great marketing return investment is good. [00:17:00] It really is a quality measure, per customer.
And the other big thing that we're gonna wanna know is how effectively are penetrating your market. Are you able to acquire a lot of customers at that value? And so those are the two big broad categories. And we'll look at both, obviously the number of acquisitions that you have over time, that's, that is your quantity measure.
But you're gonna wanna have a good forward looking sense that there's still a lot more people yet to be acquired, that you will be able to acquire. Yeah. For the quality measures. I say that's where we probably spend most of our time. Yeah. I think that big thing that we focus on is what is the value of customers after they're acquired.
And so then we can say if this is how valuable they are after they've inquired, And this is how much is being spent to acquire them, that, that allows us to be able to say yep, you're doing a good job, or, Nope, you're not doing a good job. But the reason we wanna split those two apart too, is you'll have very different customer acquisition costs by acquisition channel.
And so you inevitably one can quote, what their average C is, but that's [00:18:00] blending organic customers. Customers that have been acquired to pay channels. And one very important thing. If you're looking to establish that this company can really scale, is can they make a whole bunch of money? Do they have a high lifetime value on their pay channels?
And for them, we're gonna be looking, we're gonna be trying to focus on, measures of marginal C through pay channels and how that compares to the value of those customers after acquisi. And that's, that's a higher bar to set, but if they can really prove that out then that sort of company can acquire much Morely
[00:18:29] Andrew Michael: It's an interesting topic this as well, because I think and I've observed this at different companies before, previously at hot jar as well.
Is that the. Organic channels. As you start to hit scale sought to like account for the majority of your acquisition at some point, depending, obviously on the type of business. So I'm like generalizing the, like if you're serving SMB, mid-market generally, like at some point word of mouth would be one of the biggest drivers or it should be at least for the business and.
What ends [00:19:00] up happening when you start to break down and you split like attributional revenue to signups and trying to understand, okay, what is the payback period and the tech, when it comes from individual channels? I feel there's a bias in that, in the sense that it doesn't account for the impact and effect of marketing.
That's, unattributable in the sense of like the brand that you build, the equity that you have, the word of mouth that comes as a result of that initial marketing. And then when you start to look at. Like the customer acquisition costs on an individual channel level. Yes. Like specific channels may look extremely high, but then you have built this amazing, like inbound through word of mouth and thing that comes as a result of the one customer you get from paid brings in another three or four.
And it's a hard one, like to understand, because I remember when I first saw the numbers, I was like, shit, is that how much we spend to acquire a customer? And then I started thinking, I was like, but this. It's always been done. And this has worked extremely well. If it was the case that like it wasn't working, then it would've been cut a long time [00:20:00] ago.
How do you see it from this perspective as well? Cause I'm sure you've seen this quite a bit and seen different types of businesses.
[00:20:06] Daniel: Yeah it's a great question. I'd say, it's funny cuz in B2C we spent a lot of time in B2C. We do B2B as well. We've done a number of B2B businesses, but in B2C we tend to see the reverse that early on in a company's life, the people who adopt oftentimes they didn't need to be pushed so hard to adopt.
Yeah. So they'll often come in organically or through, some sort of referral program that the company might. But that usually at some point, you mentioned that ceiling, you hit this adoption ceiling where there's only so many people that you can acquire through through those channels.
And so the companies, they start putting out the Facebook ads, to go big. And and that's usually what causes the overall C to move up is they start moving into these more expensive channels. And the question becomes, can you prove out the economics there? I wouldn't be surprised that for some B2C B2B businesses, it's the opposite, that they go in the reverse direction, but Yeah.
That's it's interesting to hear you say that. Yeah.
[00:20:59] Andrew Michael: I [00:21:00] think it depends on the audience you're serving as also I think like enterprise definitely. You rely on these channels quite a bit and it does grow and get more and more expensive over time. But yeah. Sorry I cut you there,
[00:21:10] Daniel: but yeah the attribution question is a tough one.
And in part it's an unresolvable one because it's just, it's a tough problem. And it's almost not even a model problem. Yeah. I think that there are some great models that one can use, but it is also a big function of how good the data is. And yeah, and just, before acquisition, there's just only so much that you can tie together and.
I actually think it, maybe it could be a little bit easier in B2B and just cuz there's fewer customers and you're tracking more of the activity of, the people who may adopt, but haven't done so yet. As soon as you move to B2C, it's just terrible. There's this very limited ability to track individual people, as they're looking at exposed to different ads on one platform versus another, and then they're speaking with somebody and then they convert on the website.[00:22:00]
That person will typically be tagged as in organic acquisition, but the company spent a whole bunch of money to make it happen. Yeah. Yeah, I think, yeah, to that point it also, it speaks to the importance. I think people will tend to poo the overall C especially if you're in marketing, cuz if you market say what really matters is your marginal C and we wanna know how much you're gonna spend on that incremental customer and you know how likely it is that's gonna bring about an acquisition, ultimately these attribution issues will make that calculation very hard to do.
I'd say that the redeeming characteristic of overall C is. You're not holding back any portion of the marketing budget. You're comparing total marketing spend to total acquisitions. And maybe you should account for some lead lag between the two, but yeah. Traces you back. And in some sense, it's the most important thing, cuz ultimately if you're an investor, what you wanna know is how many customers are they gonna acquire?
What's the marketing spend gonna be? And what's revenue gonna be? You know [00:23:00] that overall C period can be really helpful for that
[00:23:01] Andrew Michael: yeah, no for sure. I think it's very helpful. Like comparing. Like different paid channels and understanding from there because you have almost like an apples to apples.
But I also have I've noticed in a few different other companies that I've been speaking to from time to time. Like an example is for example, in the crypto space now I think the audience there. Tends to be extremely, technically sophisticated, where the majority are using things like ad blockers and different types of browsers.
And so attribution's almost like impossible in that space for that specific segment in audience. And then if you're doing something certainly where you're breaking it down and trying to understand what your customer acquisition costs from specific channels, they're gonna look extremely high when an actually, maybe 20 to 50% is coming from one of those channels.
And you're unable to attribute it. So I think. The attribution I think is like gonna it's an unsolved problem. That'll probably never be solved. Like you can get a decent idea. And I think it's like the signal versus noise, like understanding, like how much should you rely on it versus how much should you ignore it?
Also,
[00:23:59] Daniel: there's a [00:24:00] question of decision orientation that we're always trying to get the best numbers what will change your decision? And that could be a slightly different question, that it could be that if you were a bit more precise, you, maybe you would've made the same decision.
And so there's a question of just how important it is to get it exactly right. We usually compute these numbers will be very upfront about what their limitations could be. And then the idea would be, what does it tell us about what we can do? And and one thing that you could do for example, is even if you knew.
There were potential attribution issues. If you had three channels that all tended to be right at the very bottom of the final or tended to. Very quick conversion or no conversion at all, then at least you can compare those three and be able to say it seems like this one is way more productive than this other one.
[00:24:48] Andrew Michael: Yeah. And that you're comparing apples to some degree as well. Exactly.
[00:24:52] Daniel: Yeah. You need to be able to you don't wanna compare the billboards to the Facebook ads yeah yeah, but.
[00:24:57] Andrew Michael: I think this is also something as like earlier in [00:25:00] my career, obsessed of trying to get the absolute clean.
Data set and make sure like the numbers were a hundred percent correct. But I think the more one thing I've learned is probably like you never have the perfect data set and you never have the most like service to get it set up, especially when you're dealing with startups. But really understanding trying to understand, what signals can you rely on versus what unreliable I think getting to that point is is quite difficult.
[00:25:24] Daniel: Yep. I agree.
[00:25:26] Andrew Michael: You, so you work as well then with evaluations for companies, like what are some of you mentioned, okay. Now how your evaluation works yourself. When it comes to the actual valuation that is set for the purchase price of these companies what are the metrics you would say are influencing that final evaluation?
The most.
[00:25:43] Daniel: Again, I'd spent my life in finance before coming back into academia and and I actually think about the valuation problem in the same way that a finance professional would. And that is that the value of a firm is driven off of its future free cash flows. And it sounds so old fashioned, but I think what goes outta [00:26:00] style comes back in style.
And here we are, in the midst of a potential, deep recession, time will tell. But I think you, if you're focusing on the cash flows, it could be a great insurance policy and that you're not getting, completely host right now. Yeah we take a traditional DCF based cash flow based valuation approach.
And the main thing that we'll do is we'll inject these customer behaviors into how we make the revenue forecast and into what the marketing spend will be. And the way I'll typically describe it is if you had a crystal ball that could tell you what the flow of customer adoptions is gonna be.
How long they're gonna stay the number of orders that they place. Again, if it's a non-subscription business and then how much they spend, then that has to give you revenue. And so it's a basic accounting identity. But now we've got the ability to take these very sophisticated, predictive models that have been cultivated over the years within the marketing science literature and use those to make more accurate forecasts of what we're gonna see in the.
So in that kind of, once we have all those models trained up, [00:27:00] it gives us the revenue forecast for free. If we also had that view as to what overall CAC has been over time and where that may be heading in the future, then that would also give us, the marketing spend into the future.
And we would then use that in conjunction kind of with your traditional. DCF approach, contribution margin, how much overhead, et cetera, that kind of get us down to net opening, profit after taxes and whatnot. Yeah, and I think it's really helpful. Not only because I think that it's the right theoretical framework for valuation, that is how firms are valued.
I really do believe that. And I think a. You know that's how finance tends to view it. But the fact that you have a model that is a hundred percent consistent with what the finance professionals use makes it more likely that your model is gonna be adopted by them. And so you're not telling them to use a completely different framework.
What you're saying is do exactly what you've been doing, but we're just gonna give you a more refined estimate of what the what those final outputs are gonna.
[00:27:58] Andrew Michael: Interesting on that as well. I'm not [00:28:00] sure. Did you see the news or was either today or yesterday that Adobe has committed to acquiring Figma?
If you're familiar with the companies, are you, I
[00:28:08] Daniel: saw that news, but I have not been following and I don't know too much about Figma. Yeah. Oh yeah.
[00:28:13] Andrew Michael: So basically I think it, it's supposed to be like one of the highest valuations on multiple of revenue ever at 50 X. My question was going to be too what do you think was going through their minds when they evaluated that company and said, okay, 50 X, as you mentioned we're going, it seems like we're going to recession now.
And yet we're seeing like one of their highest deals ever made as factor of the revenue.
[00:28:34] Daniel: Yeah, it's that's interesting. I think what I think it's very possible and feasible and sensible to, to have. Very high revenue multiples if it's merited. Yeah. I think if it's a software as a service firm and that firm is growing net revenue, from existing customers by 40% a year If it comes at very high margins.
And if the firm is very young, so there's a lot of [00:29:00] customers that they haven't acquired yet where they can be able to put up those same sort of figures. I think those would be the conditions where, you can get to pretty high multiples, 50 X. I, I don't know but certainly I think software as a service in general has been able to to earn very high multiples, maybe not 50 X.
Yeah, 10, 15, 20 X by being able to take those existing relationships, expand that business over time and and do so at a, at an 80% gross margin, just to sort of margin, you're just not gonna get B see business.
[00:29:30] Andrew Michael: Yeah, I think Adobe as well. I think they're probably eating into their market quite heavily Figma because they've built an amazing product.
Actually, my first reaction was I was a little bit sad when I heard it, cuz I was like, hope they don't ruin this. Cause it's a really good product. Not saying that Adobe's also built great products, but I think over time, like they just fell behind when it comes to other competitors in the space like Figma specifically for.
UX and UI design as well. It's amazing. Nice. I wanna make sure I ask you a couple of questions. Ask every guest they're joining the [00:30:00] show. First question is, imagine a hypothetical scenario. You're joining your company trainer. Retention's not doing great at this company. And the CEO comes to you and says, Hey, Daniel, you're in charge.
You need to turn things around in 90. What do you do? It catches. You're not gonna tell me I'm gonna go look at data or speak to customers and understand their pain points. You're just gonna pick a tactic that you've seen work previously and run with that blindly, hoping it works at this company. What would you do?
Taking away a
[00:30:29] Daniel: secret reference. Yeah. I know if I didn't have data, I feel really naked. So this will be a terrible answer. I'm sure it the operators gonna probably have much, much better answers than this, but yeah, I would say if you're non-subscription business starting up some sort of a subscription to remove frictions from your business, I've seen that work many times at the companies that we've done diligence on.
So one thing that it can often do is it can. Take your best customers, who are the ones that have higher retention. And and oftentimes those customers are not that price [00:31:00] sensitive. What they really care about is they love the product and they just wanna do more of it. And so if you can make it much easier for them, but get 'em to pay for it.
You get this stream of subscription payments from them, which obviously can be quite profitable, but you also can ramp up their order frequency. And so you get this double whammy from them. So again, not necessarily something that every company can do, but I think there is a history of companies doing that very well.
[00:31:25] Andrew Michael: Yeah. I think Amazon prime comes to mind when you mention that specific example. Nice. What's one thing that, you know today about churn and retention that you wish you knew when you got started with your career?
[00:31:36] Daniel: I'd say, the great thing is there's tremendous variability across companies, even companies within the same category.
And so it really is just a very useful measure to focus on because there are all these differences. If every company had the same general profile Then there's not a whole lot of room to pick winners and losers based on it, but but we definitely don't see that. Yeah, so I say, yeah, that's that's something [00:32:00] that I didn't really have a sense for the magnitude of the heterogeneity until I started looking at the data.
[00:32:05] Andrew Michael: Yeah, it is interesting. It's actually one of the reasons we always try stray away from asking you specific numbers on the show because I think you end up leaving people with the wrong idea. Cause it does very drastically depending on the space, the segment, the audience you're going after your product.
So yeah, I definitely see that point there. Nice Daniel, it's been a pleasure hosting you today. Is there any final thoughts that you wanna leave the listeners with before you drop off today? How can they keep up to speed with your.
[00:32:31] Daniel: The best places to keep up with me are on LinkedIn and Twitter.
And so I write very frequently on on both platforms. So yeah, happy to give the handle for Twitter, but it's a D underscore MCR, M C a R. And then on LinkedIn, think it be just Google for Daniel McCarthy, Emory, hopefully it'll pull up, but yeah, I live and breathe this and some I'm writing about it.
Very often I also teach a course it's called customer lifetime valuation. It's all about exactly what we've been talking [00:33:00] about. So I really am the ultimate one trick pony but it's a really fun trick. So I don't mind .
[00:33:06] Andrew Michael: Yeah, we'll definitely make sure to leave that in the show notes. If you're listening and you wanna catch up later, you'll be able to find that there as well.
Thanks so much for joining and wish you best of luck going forward. Yeah. Thanks for having
[00:33:17] Daniel: me. This has been great. Cheers.
Comments

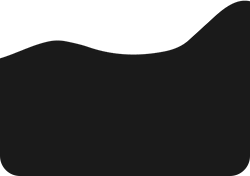
Daniel McCarthy
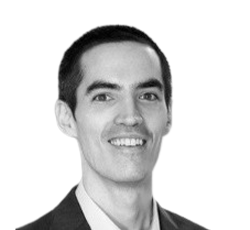
A new episode every week
We’ll send you one episode every Wednesday from a subscription economy pro with insights to help you grow.
About
The show
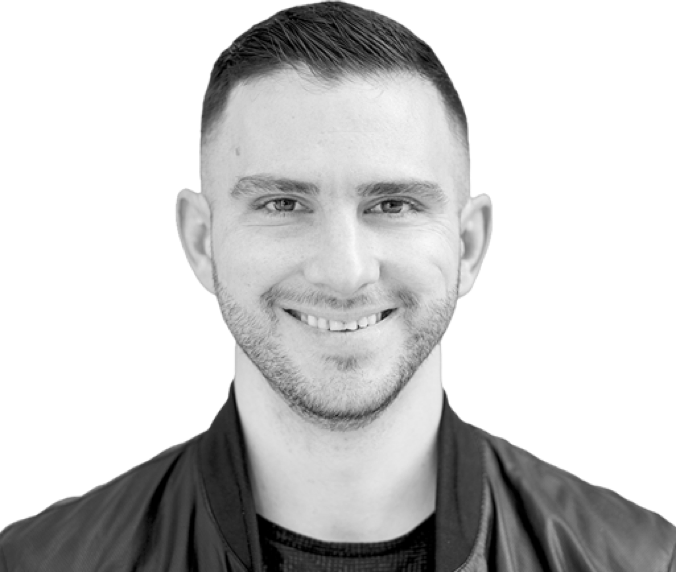
My name is Andrew Michael and I started CHURN.FM, as I was tired of hearing stories about some magical silver bullet that solved churn for company X.
In this podcast, you will hear from founders and subscription economy pros working in product, marketing, customer success, support, and operations roles across different stages of company growth, who are taking a systematic approach to increase retention and engagement within their organizations.